The Power of Image Automation in Modern Business
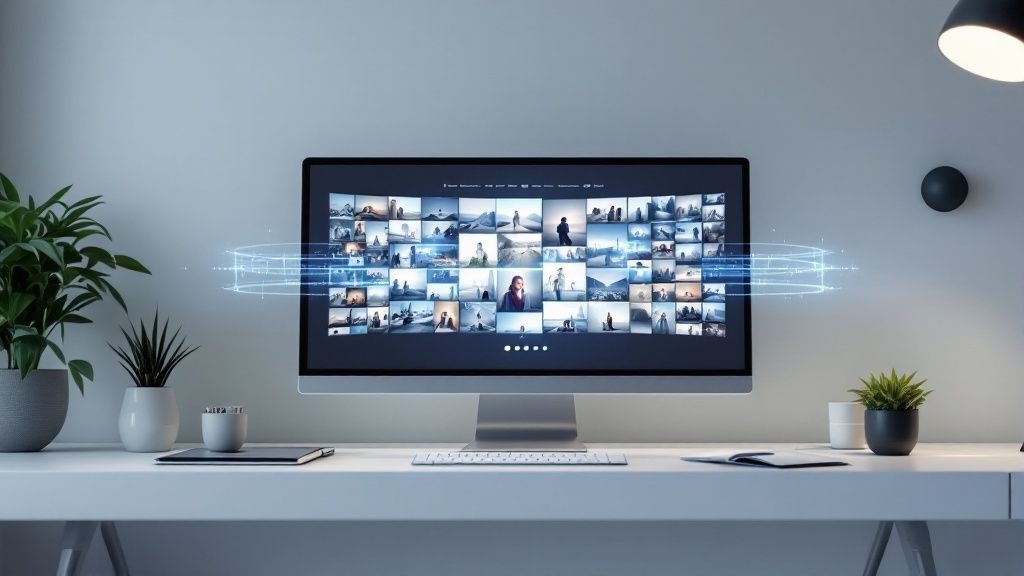
Image automation is reshaping business operations, providing significant advantages over traditional manual image processing. This shift affects how businesses handle visual data, from simple adjustments to complex analysis.
One primary benefit is automating repetitive tasks, freeing up valuable employee time for more strategic work. This allows companies to prioritize growth and innovation.
This rising need for automated image processing solutions is a major market driver. The global image recognition market is a prime example.
In 2022, the market was valued at roughly $28.3 billion.
Projections indicate a substantial increase to $126.8 billion by 2032.
This represents a robust compound annual growth rate (CAGR) of 16.5% between 2023 and 2032.
Factors contributing to this growth include advancements in deep learning and the expanding use of image automation across diverse industries. For more in-depth market statistics, you can find more detailed statistics here.
Efficiency Gains Through Automation
Automated image processing leads to significant improvements in business efficiency.
This increased efficiency often results in substantial cost savings.
By automating tedious manual tasks, businesses can lower labor costs and better allocate resources.
Imagine a company processing thousands of images every day. Automating tasks like resizing, tagging, and sorting can dramatically reduce processing time and free up employees for higher-value work.
Enhancing Accuracy and Consistency with Image Automation
Human error is inevitable in manual processes. Image automation helps minimize these errors, improving the accuracy of tasks like image analysis and data extraction.
Automated systems also ensure consistency in image processing, regardless of data volume or complexity.
This consistency is vital for businesses needing standardized image processing across various departments or locations. Maintaining this standard is particularly important for fields like medical imaging and manufacturing quality control.
To illustrate the advantages of automated image processing, let's look at a comparison table.
The following table compares manual and automated image processing across several key performance metrics.
Manual vs. Automated Image Processing Comparison | Performance Metric | Manual Processing | Automated Processing | Improvement Factor | |---|---|---|---| | Speed (images/hour) | 100 | 10,000 | 100x | | Error Rate (%) | 5 | 0.1 | 50x | | Consistency (%) | 90 | 99.9 | ~10% | | Cost per Image ($) | 0.50 | 0.01 | 50x |
As you can see, automated image processing provides significant improvements across all metrics. The speed increase is particularly noteworthy, allowing businesses to process images much faster. The reduction in error rate and increased consistency also lead to higher quality results. Finally, the substantial cost savings per image can have a significant impact on a business's bottom line.
The Future of Visual Data
The future of image automation is deeply connected to advances in Artificial Intelligence (AI) and Machine Learning.
These technologies are constantly expanding the possibilities of image analysis and processing.
As AI algorithms become more advanced, businesses can anticipate even higher levels of automation, accuracy, and efficiency in their image-based workflows. This translates to better decision-making, improved customer experiences, and a stronger competitive advantage.
From Pixels to Productivity: The Evolution Journey
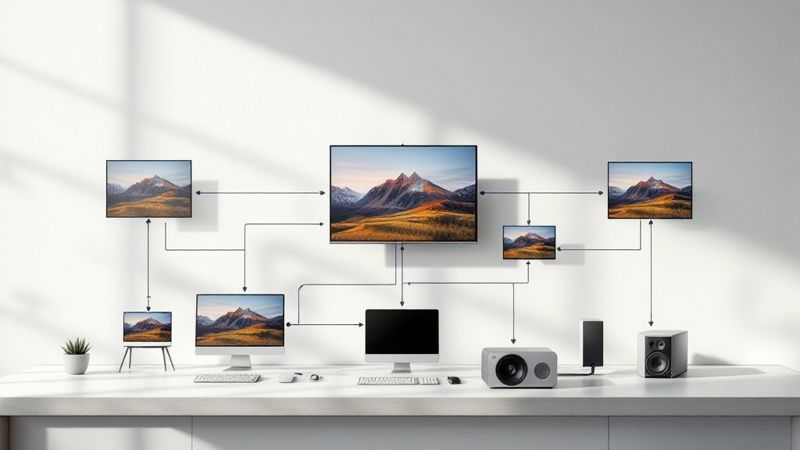
The story of image automation is one of constant progress.
Initially, this technology focused on simple digitization.
Now, thanks to artificial intelligence, it tackles complex image analysis and manipulation.
This wouldn't be possible without major leaps in algorithms and computing power, the very foundations of today's advanced image automation systems.
The Rise of Document Image Processing
A critical development was document image processing (DIP).
Throughout the early and mid-1980s, minicomputer-based DIP systems from companies like Canon, Kodak, and Toshiba were the norm.
These converted paper documents to digital images.
A significant breakthrough was the 1985 release of the Discus 1000, the first microcomputer-based optical filing system.
DIP systems also integrated optical character recognition (OCR), making text searchable and editable, and revolutionizing document management.
Early OCR could only recognize single fonts. Today's OCR handles multiple fonts and languages, dramatically boosting efficiency. Learn more about this evolution.
The Impact of AI and Machine Learning
The advent of AI and machine learning was a game-changer.
These technologies enabled image automation to move beyond basic tasks to more intricate analyses. For instance, image recognition algorithms now identify objects, faces, and even emotions within images.
Furthermore, machine learning allows these systems to learn and improve over time, resulting in greater accuracy and efficiency.
Visuals play a vital role in automation, providing feedback and guiding the process.
This is especially true for personalized image creation, a core aspect of platforms like OKZest. For more on visual support, check out these visual support strategies.
The Future of Image Automation
The future of image automation holds even greater potential. As AI and machine learning continue to advance, we can anticipate systems that analyze images with increased precision and speed.
This will empower businesses to automate more complex tasks, leading to higher productivity and significant cost savings.
Moreover, integrating image automation with technologies like augmented reality and virtual reality will unlock new avenues for innovation. The possibilities are truly vast, impacting diverse industries from healthcare and manufacturing to marketing and entertainment. This continued growth and evolution heralds exciting changes in how we interact with and utilize visual data.
Building Blocks: What Makes Image Automation Work
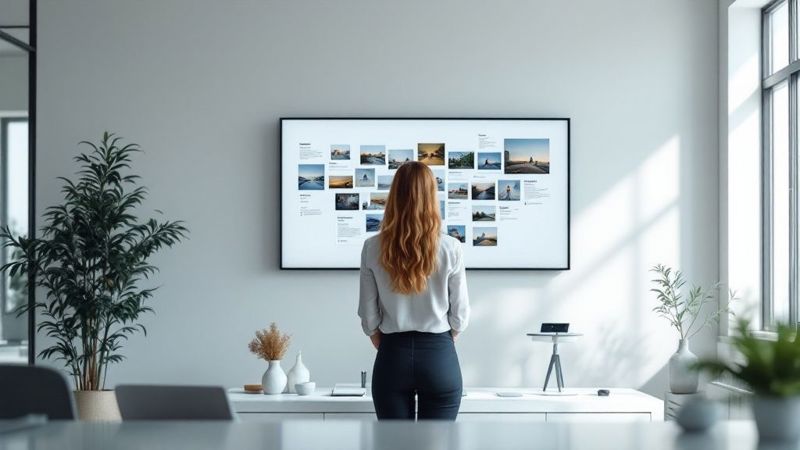
Let's explore the core components behind effective image automation systems.
Understanding these elements is crucial for building solutions that deliver real results.
This involves examining key aspects, from initial image capture to the algorithms that unlock valuable information.
Essential Components of Image Automation
Image automation relies on hardware and software working in concert. This combined approach allows for efficient processing and analysis of visual information.
*
Image Capture: High-quality images are the bedrock of any successful image automation system.
This often involves using specialized cameras and scanners designed for specific tasks, such as industrial inspection or medical imaging.
*
Preprocessing:
Captured images often require preprocessing steps. These can include noise reduction, color correction, and resizing to prepare the images for analysis.
This crucial stage improves data quality and the effectiveness of later processing.
*
Feature Extraction: This stage focuses on identifying key characteristics within an image.
These features, like edges, corners, and textures, are fundamental for understanding the image content.
They're crucial for tasks like object recognition.
*
Classification:
Algorithms are used in this stage to categorize or label images based on their extracted features. This can include identifying objects, detecting defects, or classifying medical images for diagnosis.
Hardware and Software Considerations
Choosing the right hardware and software is essential for successful image automation.
The selection process involves balancing factors like processing power, compatibility, and scalability.
*
Processing Power: Image processing can be computationally demanding, especially when dealing with large datasets or complex algorithms. Carefully selecting hardware with sufficient processing power is vital.
*
Compatibility:
Ensuring seamless compatibility between hardware and software components is key to a smooth, integrated workflow.
This is particularly important when connecting with pre-existing systems.
*
Scalability: As your data grows, your system must be able to handle the increasing volume.
Choosing scalable solutions for both hardware and software is crucial for long-term success.
To illustrate the key elements of image automation systems, let's examine the following table:
The table below provides a breakdown of the essential hardware and software elements required for implementing image automation solutions.
Component Type | Function | Technology Options | Selection Considerations |
---|---|---|---|
Image Capture | Acquiring visual data | Cameras, scanners, mobile devices | Resolution, speed, lighting conditions |
Preprocessing | Enhancing image quality | Noise reduction, color correction, resizing | Algorithm selection, computational resources |
Feature Extraction | Identifying key image characteristics | Edge detection, texture analysis | Algorithm complexity, feature relevance |
Classification | Categorizing images | Machine learning models, deep learning networks | Accuracy, training data, computational demands |
This table highlights the interconnectedness of each component and the important considerations when selecting technologies for each stage of the process.
Effective image capture provides the foundation, while preprocessing and feature extraction prepare the data for accurate classification.
For businesses interested in using images for personalized marketing, automated solutions offer many advantages.
See our guide on How Personalized Image Software Can Revolutionize Your Marketing Strategy for more information. Investing in robust hardware and software is essential for any successful image automation strategy, enabling efficient processing and adaptation to growing data volumes.
Image Automation Success Stories Across Industries
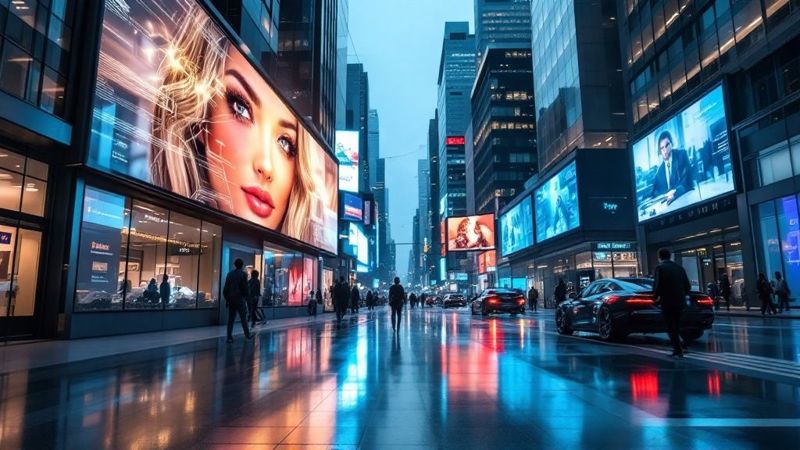
Real-world examples showcase the impact of image automation across diverse sectors.
These success stories illustrate how businesses are using this technology to tackle challenges and improve their competitive advantage. Let's delve into a few examples of image automation in practice.
Healthcare: Enhanced Diagnosis and Treatment
Image automation has become essential in healthcare.
AI-powered systems, for instance, can analyze medical images like X-rays and CT scans. This helps detect diseases such as cancer earlier and with greater accuracy compared to traditional methods, leading to faster intervention and better patient outcomes.
Image automation also streamlines administrative work, including processing patient records and managing medical images.
This frees up healthcare professionals to dedicate more time to direct patient care.
Retail: Optimized Inventory and Customer Experience
Retailers leverage image automation for efficient inventory management.
Computer vision systems can track stock levels in real time.
These systems identify low-stock items and automatically initiate restocking orders.
This approach minimizes waste, prevents stockouts, and enhances overall supply chain efficiency.
Image automation also contributes to a more personalized customer experience.
Some retailers, for example, use AI to recommend products aligned with customer preferences. This can boost conversion rates and improve customer satisfaction.
For more insights into using visuals in marketing, see How to master dynamic email images to boost email marketing.
Manufacturing: Improved Quality Control and Defect Detection
Image automation contributes significantly to quality control in manufacturing.
Automated visual inspection systems identify defects with remarkable precision.
This reduces errors and improves product consistency, leading to less waste, fewer product recalls, and a stronger brand reputation.
Real-time monitoring of production lines is another advantage.
Image automation systems can detect potential problems early, preventing them from escalating into larger, more costly issues. This helps avoid downtime and maintain efficient production.
Security: Advanced Threat Detection and Surveillance
Modern security systems rely heavily on image automation.
AI-powered systems analyze video feeds to detect suspicious activity, bolstering public safety and preventing security breaches.
Facial recognition technology, for example, can identify potential threats in crowded places. Automated surveillance systems can monitor remote locations for unauthorized access.
These systems enable rapid responses to potential security incidents, reducing risks and creating safer environments.
Document Management: Streamlined Data Extraction and Processing
Businesses dealing with high volumes of documents find significant value in image automation. Automated systems can extract information from documents like invoices and contracts. This reduces the need for manual data entry, increasing both accuracy and speed.
Workflows become more efficient, processing times are reduced, and the risk of human error is minimized.
Employees can then focus on more strategic tasks, optimizing the use of human resources. AI-powered image processing tools are increasingly important in these applications.
The market for these tools was valued at approximately $1.4 billion in 2024 and is projected to reach $9.42 billion by 2034, growing at a CAGR of 21.00%.
Learn more about AI-powered image processing tools.
For further information on website optimization strategies, consider consulting with Technical SEO services.
Your Image Automation Implementation Blueprint
This practical, step-by-step guide helps you implement image automation within your organization.
Drawing from successful implementations across various industries, this blueprint offers a clear roadmap for the entire process.
Identifying Opportunities and ROI
First, pinpoint areas where image automation offers the most value.
Look for repetitive, time-consuming tasks, or those prone to human error.
Calculating the potential return on investment (ROI) involves estimating the cost savings and efficiency gains.
For instance, automating image resizing and tagging for e-commerce product photos can significantly reduce labor costs and improve listing speed. This targeted approach ensures your automation aligns with business goals.
Build vs. Buy: Making the Right Choice
After identifying opportunities, decide whether to build a custom image automation solution or buy an existing platform. Building offers more control but requires significant development resources.
Buying a platform is typically faster and more cost-effective, but might require compromises in functionality.
Consider your needs, technical expertise, and budget. If you primarily need to personalize images for email marketing, a platform like OKZest might be a more practical choice.
Technology and Vendor Selection
Whether building or buying, evaluate the technologies and vendors. Look for solutions aligning with your long-term strategy and scalability needs.
Consider factors like ease of integration, compatibility with existing systems, and vendor support.
Careful assessment helps avoid costly mistakes and ensures smooth implementation.
Preparing Your Data Infrastructure
Image automation relies on access to high-quality data.
Ensure your data infrastructure supports your automation initiatives.
This includes organizing your image library, implementing data quality control, and establishing secure data storage and access protocols. A well-prepared data infrastructure optimizes your automation systems.
Assembling Your Team and Driving Adoption
A cross-functional team is crucial for successful implementation. Involve stakeholders from different departments to ensure the solution meets everyone's needs. Clearly define roles and responsibilities, and promote open communication.
For email marketing, you might find this helpful: Streamline Your Email Marketing with Image Automation.
Address any resistance to change with effective communication and training.
This fosters buy-in and ensures smooth adoption.
Measuring Success and Demonstrating Impact
Define metrics to measure the success of your image automation. Track key performance indicators (KPIs) such as processing time, error rates, and cost savings.
Regularly monitor these metrics and adjust as needed.
This data-driven approach demonstrates the impact of automation and secures support for future projects.
Consistent measurement and analysis optimize your systems.
Governance and System Integrity
Establish a governance framework to ensure the long-term integrity of your systems. This includes implementing data security protocols, establishing quality control procedures, and conducting regular audits.
This maintains data accuracy, protects against security breaches, and ensures reliable results over time. A robust governance framework protects your investment and maximizes its long-term value.
Navigating Image Automation Challenges Head-On
Implementing image automation offers significant advantages, but it's not without its challenges. Let's explore some common hurdles and how to overcome them.
Data Quality Issues
Maintaining high data quality is crucial.
Insufficient training data can limit the effectiveness of machine learning models.
If the dataset doesn't accurately represent real-world scenarios, the system's performance will suffer.
For example, an image recognition system trained only on clear images might struggle with blurry images in practice.
Biased datasets present another challenge.
If training data overrepresents certain characteristics, the system might amplify these biases.
A facial recognition system trained primarily on images of one demographic might be less accurate at recognizing others.
This underscores the need for diverse and representative datasets.
Technical Limitations
Technical limitations can also hinder image automation.
Handling edge cases – unusual scenarios not in the training data – can be difficult. An automated system designed to identify standard product packaging might struggle with damaged packages.
Variations in the environment, such as lighting or camera angles, also pose challenges.
A system trained under specific lighting conditions might fail when those conditions change.
Overcoming this often requires robust algorithms.
Integration Challenges
Integrating image automation with legacy systems can be complex.
Older systems may lack compatibility with required data formats.
This can necessitate significant rework or custom integration solutions, increasing implementation time and cost.
Connecting a modern image recognition system to an older inventory system might require custom APIs.
Privacy and Security Concerns
Privacy and security are paramount.
Handling sensitive image data requires strict adherence to regulations and best practices.
Non-compliance can lead to legal and reputational damage.
Systems processing medical images must comply with HIPAA regulations. Systems using facial recognition technology must address privacy concerns. A robust security framework is essential, especially when handling personally identifiable information.
Organizational Resistance
Finally, organizational resistance can hinder adoption.
Employees unfamiliar with image automation might be hesitant to embrace new workflows.
Addressing these concerns requires clear communication, training, and ongoing support.
Demonstrating the benefits of image automation – efficiency gains, improved accuracy, and more rewarding tasks – is key to fostering buy-in.
Ready to automate your image creation process? Start personalizing your images with OKZest today!